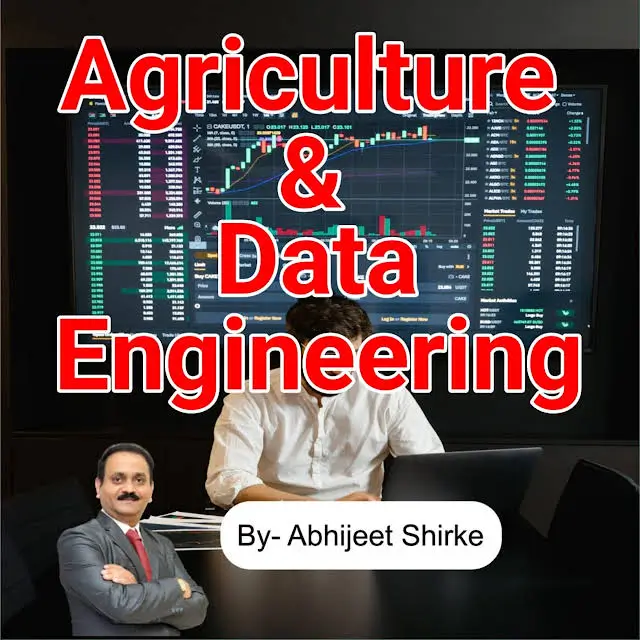
Indian Agriculture and Data Engineering has the potential to intersect and create significant benefits for the agricultural sector. Data engineering involves high volume data collection, storage, processing, and analysis. Agriculture in India is a crucial sector of the country’s economy, employing a significant portion of the population. Here are some ways in which data engineering can contribute to Indian agriculture
Agriculture has been the backbone of human civilization for millennia, providing the food and resources necessary for societal development. In recent years, the integration of advanced technologies into agricultural practices has revolutionized the sector, bringing about significant improvements in productivity, sustainability, and efficiency. One such technological advancement is data engineering, which plays a critical role in transforming traditional agricultural methods. This article explores the concept of data engineering, its importance in agriculture, and its specific relevance to Indian agriculture, highlighting how it can contribute to the Indian GDP and overall economic growth.
Understanding Data Engineering
Data engineering is the process of designing, constructing, and maintaining systems and architectures that collect, store, and process large volumes of data. It involves various activities, including data collection, data storage, data transformation, and data analysis. The goal of data engineering is to ensure that data is accessible, reliable, and ready for analysis by data scientists and analysts.
Key Components of Data Engineering
1. Data Collection: Gathering data from various sources, including sensors, satellites, databases, and manual inputs.
2. Data Storage: Utilizing databases and data warehouses to store large volumes of structured and unstructured data efficiently.
3. Data Transformation: Cleaning, transforming, and aggregating data to make it usable for analysis.
4. Data Integration: Combining data from different sources to provide a unified view.
5. Data Pipeline: Automating the flow of data from collection to storage and analysis.
6. Data Governance: Ensuring data quality, security, and compliance with regulations.
Importance of Agriculture and Data Engineering
Data engineering is crucial in modern agriculture for several reasons:
- Precision agriculture Enables farmers to use data-driven techniques to optimize crop yields, reduce waste, and minimize environmental impact. By analyzing data from soil sensors, weather stations, and drones, farmers can make informed decisions about planting, irrigation, fertilization, and harvesting.
- Resource Management: Helps in efficient management of water, soil, and other agricultural inputs. Data on weather patterns, soil moisture levels, and crop health can be used to optimize resource usage, reduce costs, and improve sustainability.
- Supply Chain Optimization: Facilitates better planning and management of the agricultural supply chain. Data on production levels, market demand, and logistics can help in reducing post-harvest losses and ensuring timely delivery of produce to markets.
- Risk Management: Provides tools for managing risks associated with weather, pests, and diseases. By analyzing historical data and real-time information, farmers can anticipate and mitigate potential threats to their crops.
- Market intelligence: enables access to market trends, pricing information, and consumer preferences. This helps farmers make strategic decisions about what crops to plant and when to sell their produce.
Data Engineering and Indian Agriculture
India is predominantly an agrarian economy, with agriculture accounting for around 17–18% of the GDP and employing nearly 50% of the workforce. Despite its critical importance, Indian agriculture faces numerous challenges, including low productivity, inefficient resource use, fragmented supply chains, and vulnerability to climate change. Data engineering offers a promising solution to these challenges by enabling the adoption of smart farming practices.
Enhancing Productivity
Data engineering can significantly enhance agricultural productivity in India. By leveraging data from various sources, including satellites, sensors, and drones, farmers can gain insights into soil health, crop growth, and pest infestations. Precision farming techniques, supported by data engineering, allow for precise application of fertilizers, pesticides, and water, leading to higher yields and reduced input costs. For instance, data from soil sensors can indicate nutrient deficiencies, allowing farmers to apply the right type and amount of fertilizer.
Optimizing Resource Use
Water scarcity is a major issue in Indian agriculture, with irrigation accounting for a significant portion of water usage. Data engineering can help optimize water use through smart irrigation systems that monitor soil moisture levels and weather forecasts to provide the right amount of water at the right time. This not only conserves water but also enhances crop health and yields.
Improving Supply Chain Efficiency
Indian agriculture suffers from inefficiencies in the supply chain, leading to significant post-harvest losses. Data engineering can streamline the supply chain by providing real-time data on production, demand, and logistics. This enables better planning and coordination among various stakeholders, reducing delays and ensuring that produce reaches markets in optimal condition. For example, data analytics can predict demand trends, allowing farmers to time their harvests to meet market demand and reduce waste.
Risk Management and Climate Resilience
Climate change poses a significant threat to Indian agriculture, with an increasing frequency of extreme weather events such as droughts and floods. Data engineering can aid in risk management by providing early warning systems and predictive analytics. Historical weather data, combined with real-time monitoring, can help in forecasting adverse weather conditions and advising farmers on appropriate measures to protect their crops. Additionally, data on pest and disease outbreaks can enable timely interventions, reducing crop losses.
Empowering Farmers with Information
Access to market information is crucial for farmers to make informed decisions about what to grow and when to sell. Data engineering can provide farmers with insights into market prices, demand trends, and consumer preferences. Mobile applications and digital platforms can disseminate this information to farmers, enabling them to optimize their production and marketing strategies. For example, farmers can use price forecasting models to decide the best time to sell their produce, maximizing their income.
Boosting the Indian Economy through Data Driven Agriculture
The integration of data engineering into Indian agriculture has the potential to boost the economy in several ways:
- Increased Agricultural Output: By enhancing productivity and efficiency, data engineering can lead to higher agricultural output. This not only contributes to food security but also increases the income of farmers, leading to improved living standards and reduced poverty.
- Export Competitiveness: Higher quality and consistent agricultural produce can boost India’s competitiveness in international markets. Data-driven agriculture can help in meeting quality standards and reducing post-harvest losses, making Indian produce more attractive to global buyers.
- Job Creation: The adoption of data engineering and related technologies in agriculture can create new job opportunities in rural areas. These include roles in data collection, analysis, and technology maintenance, as well as in the development and deployment of smart farming solutions.
- Sustainable Development: Data engineering promotes sustainable agricultural practices by optimizing resource use and minimizing environmental impact. This aligns with India’s goals for sustainable development and can attract investment in green technologies.
- Policy Formulation: Accurate and timely data is crucial for effective policy formulation. Data engineering can provide policymakers with insights into agricultural trends, helping them design targeted interventions and allocate resources more efficiently.
Data driven decision making:
By collecting and analyzing agricultural data, such as weather patterns, soil conditions, crop yields, and market trends, farmers can make more informed decisions. Data engineering techniques can help process and interpret this data, enabling farmers to optimize their farming practices, maximize yields, and minimize risks.
Precision Agriculture
Data engineering can support precision agriculture techniques, where farmers use data to make precise and targeted decisions for irrigation, fertilizer application, and pest control. By leveraging data from sensors, satellites, and other sources, farmers can optimize resource utilization and minimize environmental impact.
Market analysis and forecasting:
Data engineering can aid in analyzing market trends, demand patterns, and commodity prices. After data collection from various sources and its processing, farmers and policymakers can make informed decisions regarding crop selection, production planning, and marketing strategies.
Crop disease detection and management:
Data engineering can facilitate the development of systems for early detection and management of crop diseases. After integrating data from satellite imagery, weather patterns, and disease databases, farmers can identify potential outbreaks, take preventive measures, and mitigate the spread of diseases.
Supply chain optimization:
Data engineering techniques can help optimize the agricultural supply chain by streamlining processes, improving logistics, and reducing wastage. Supply chain inefficiencies identified and addressed based on transportation, storage, and market demand data collection and analysis.
Farm Management Systems
Data engineering can enable the development of farm management systems that integrate data from various sources, such as farm machinery, sensors, and weather stations. These systems can provide real-time insights into crop health, soil conditions, and equipment performance, helping farmers make proactive decisions.
Government policies and subsidies:
Data engineering can support policymakers in formulating effective agricultural policies and subsidy programs. By analyzing data on agricultural productivity, resource utilization, and market dynamics, policymakers can identify areas of improvement and design targeted interventions to support farmers.
Case Studies and Examples
Precision Agriculture in Punjab
Punjab, known as the “Granary of India,” has been at the forefront of adopting precision agriculture techniques. The use of data engineering in Punjab has led to significant improvements in crop yields and resource use efficiency. For instance, farmers in Punjab have utilized data from soil sensors to tailor their fertilization practices, resulting in higher yields and reduced fertilizer usage. Additionally, the use of satellite imagery and weather data has enabled better irrigation management, conserved water, and enhanced crop health.
Smart Irrigation in Maharashtra
Maharashtra, a state prone to droughts, has benefited from smart irrigation systems supported by data engineering. Farmers in Maharashtra have adopted drip irrigation systems that are connected to soil moisture sensors and weather forecasting models. These systems automatically adjust the amount of water supplied based on real-time data, leading to significant water savings and improved crop yields. The success of these initiatives has encouraged wider adoption of smart irrigation practices across the state.
Market Linkages in Andhra Pradesh
The state of Andhra Pradesh has implemented digital platforms that connect farmers with markets, providing real-time information on prices, demand, and logistics. These platforms, supported by data engineering, have empowered farmers to make informed decisions about what to grow and when to sell. As a result, farmers in Andhra Pradesh have experienced better market access and higher incomes. The use of data analytics has also helped in reducing post-harvest losses by improving supply chain coordination.
Challenges and the Way Forward
While data engineering holds great promise for Indian agriculture, several challenges need to be addressed to fully realize its potential:
1. Digital Infrastructure: The lack of robust digital infrastructure in rural areas is a significant barrier to the adoption of data-driven agriculture. Investments in internet connectivity, data storage, and processing capabilities are essential.
2. Data Literacy: Farmers need to be educated and trained in the use of data and digital tools. Extension services and training programs can play a crucial role in building data literacy among farmers.
3. Data Privacy and Security: Ensuring the privacy and security of agricultural data is vital to gaining the trust of farmers. Clear regulations and standards for data governance need to be established.
4. Interoperability: The integration of data from various sources requires interoperability between different systems and platforms. Standardization of data formats and protocols can facilitate seamless data integration.
5. Scalability: Solutions need to be scalable to cater to the diverse and large-scale nature of Indian agriculture. Customizable and adaptable technologies can address the unique needs of different regions and crops.
Data engineering has the potential to transform Indian agriculture, address key challenges, and drive economic growth. By enhancing productivity, optimizing resource use, improving supply chain efficiency, and empowering farmers with information, data engineering can contribute significantly to the Indian GDP.
The successful integration of data engineering into agriculture requires concerted efforts from the government, private sector, and agricultural communities. Investments in digital infrastructure, education, and training, along with robust data governance frameworks, can pave the way for a data-driven agricultural revolution in India. As India moves towards becoming a digital economy, leveraging data engineering in agriculture can ensure sustainable development, food security, and improved livelihoods for millions of farmers.
To harness the potential of data engineering in Indian agriculture, it is crucial to address challenges such as data accessibility, connectivity, and digital literacy among farmers. Collaborations between government agencies, research institutions, and technology companies can play a vital role in developing robust data engineering solutions tailored to the specific needs of Indian agriculture.